Introspect#
Introspection is the examination of the activations of a neural network as data passes through. Introspecting networks and data can help improve an ML pipeline’s efficiency, robustness, and fairness.
DNIKit Introspectors
are the core algorithms of DNIKit.
You can see all available introspectors on the following pages:
As noted previously, DNIKit uses evaluation, and so
each Introspector
has an
.introspect()
method which will trigger the
Producers
to generate data and the
pipelines
to consume and process it. This is demonstrated in
the diagram below.

Exploring Results#
To explore the result of an introspection,
DNIKit’s network introspectors (PFA, IUA) have a .show()
method built-in, that
can be run in a Jupyter notebook to view the results live.
For these show methods, the result of the .introspect()
call should be passed in
as the first argument.
For instance, an example for IUA:
iua_result = IUA.introspect(producer, batch_size=64) # introspect!
# Show inactive unit analysis results (with default params)
IUA.show(iua_result)
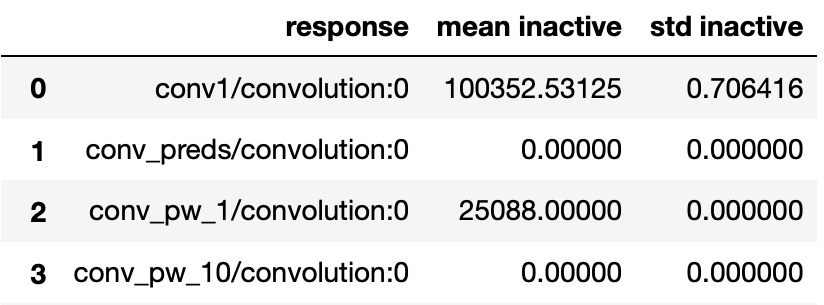
The results of DNIKit data introspectors can be visualized and explored in different manners. If the data introspectors are run as part of the Dataset Report, the introspection results may be directly fed to and explored interactively with the Symphony UI. If the introspector is run outside of the Dataset Report, the DNIKit notebook examples show one of many possible ways each result may be visualized.
Best Practices#
Preparing Inputs for Introspectors#
There are various ways in which DNIKit introspection can be tailored for different use cases. Here are some common things for users to think about:
Which intermediate layer(s) to extract model responses from
Whether to attach metadata to batches (e.g., labels and unique IDs), for instance to refer back to the original data samples with a unique identifier
Whether to pool responses or reduce dimensionality before running model responses through the introspector
Selecting Model Responses#
To use an introspector, typically certain layer(s) of the network model are used
rather that using the final outputs (or predictions). These layer names can be
provided as input, and thus requires finding the correct layer names. It’s possible to
inspect a dictionary of responses with the
response_infos
method:
model = ... # load model here, e.g. with load_tf_model_from_path
response_infos = model.response_infos
DNIKit also provides a utility function for finding
input layers from a Model
model = ... # load model here, e.g. with load_tf_model_from_path
input_layers = model.input_layers
input_layer_names = list(input_layers.keys())
Caching responses from pipelines#
When running DNIKit in a Jupyter notebook, a good rule of thumb is to
cache
(temporarily store on disk) responses at a point in the pipeline where
it doesn’t make sense to re-run every time the pipeline is processed (e.g. via introspect).
This can be done by adding a Cacher
as a
PipelineStage
. For instance:
from dnikit_tensorflow import TFDatasetExamples, TFModelExamples
from dnikit.base import pipeline
from dnikit.introspectors import Familiarity
from dnikit.processors import ImageResizer, Cacher
# Load data, model, and set up batch pipeline
cifar10 = TFDatasetExamples.CIFAR10()
mobilenet = TFModelExamples.MobileNet()
response_producer = pipeline(
cifar10,
ImageResizer(pixel_format=ImageResizer.Format.HWC, size=(224, 224)),
mobilenet()
# Cache responses from MobileNet inference
Cacher()
)
In this code, the CIFAR10 dataset will only be pulled through the MobileNet
model a single time, regardless of how many times response_producer
is used later.
The response_producer
can then be fed to various introspectors
or perform post-processing by creating new pipelines
using response_producer
as the producer
. It is on the user
to decide if caching will use significant space on their machine, and if it is worth the speed-up.
For instance, caching a single model response per data sample (caching after model inference)
will take up less space than caching large video data samples before model inference.
For a list of available pipeline stage objects, see the Batch Processors section.